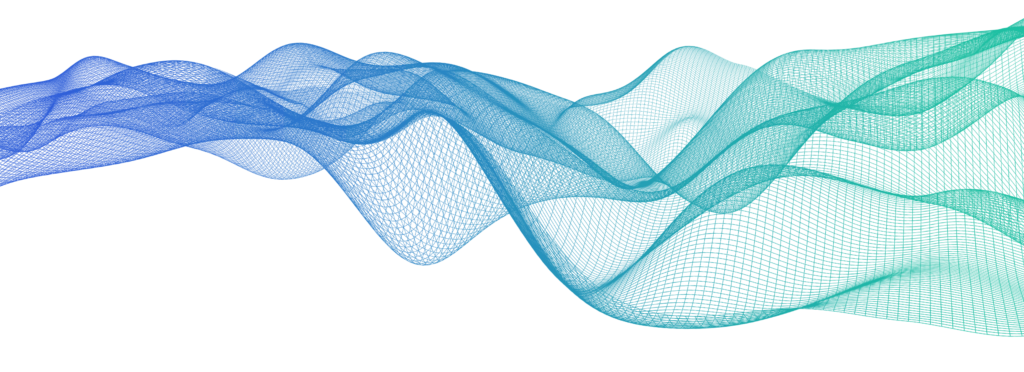
Navigating the Ethical Frontier: Ensuring Safe and Responsible AI
Welcome to an essential exploration for data scientists, AI enthusiasts, and ethical technologists!
I’m excited to introduce this comprehensive blog series focused on the pivotal topics of AI safety, reliability, and ethics.
As AI continues to integrate into every facet of our lives, understanding and implementing responsible AI practices is not just an option—it’s a necessity.
Why This Series Matters
In the race to innovate with AI, we often overlook the significant risks and ethical challenges that accompany this powerful technology.
This series is designed to fill that gap, equipping you with the insights and tools needed to create AI systems that are not only advanced but also safe, fair, and trustworthy.
What We’ll Explore
Throughout this series, we’ll dive deep into seven critical areas of AI safety and ethics:
- Fairness, Bias Detection, and Mitigation: Unearth hidden biases in your data and models, and learn effective techniques to ensure fairness across diverse demographic groups.
- Model Explainability and Interpretability: Unveil the inner workings of black-box models, making them more transparent and their decisions easier to justify.
- Reliability and Robustness: Explore advanced strategies, including adversarial training, to enhance the reliability and robustness of machine learning models.
- Ethical Considerations: Explore the critical ethical challenges in machine learning, focusing on bias detection, fairness, transparency, and accountability.
- Adversarial Robustness: Understand how to safeguard your models against malicious attacks and maintain their reliability in adversarial settings.
- Privacy-Preserving Machine Learning: Discover techniques to protect data privacy while leveraging AI, including differential privacy, federated learning, and secure multi-party computation.
- Scalable Oversight of AI Systems: Learn methods to maintain effective human control over increasingly complex AI, from recursive reward modeling to adaptive interaction protocols.
- Ethical AI Development: Explore frameworks and best practices for embedding ethical considerations throughout the AI development lifecycle.
What Makes This Series Unique
This series stands out by going beyond theoretical discussions to offer:
✓ Practical Python code examples for implementing AI safety measures.
✓ Real-world case studies that highlight the importance of these concepts in practice.
✓ Industry-specific discussions on the implications of AI safety and ethics.
✓ Insights into emerging trends in the AI ethics landscape.
Whether you’re an experienced data scientist, an AI researcher, or a business leader deploying AI solutions, this series will provide you with actionable insights and practical tools to navigate the intricate world of AI ethics and safety.
Why This Matters Now
As AI systems gain influence, the need for responsible AI practices becomes increasingly urgent. From facial recognition in law enforcement to AI-driven hiring processes, the real-world impact of AI is profound and wide-reaching.
By mastering the concepts in this series, you’ll be prepared to:
✓ Build trustworthy and reliable AI systems that users and stakeholders can depend on.
✓ Mitigate risks associated with AI deployment in various environments.
✓ Contribute to the responsible evolution of AI technology in your field.
Join the Journey Towards Responsible AI
I invite you to embark on this vital journey through AI safety and ethics. Whether your goal is to enhance model reliability, ensure fairness, or gain a deeper understanding of AI’s ethical dimensions, this series is tailored to provide the guidance you need.